Image Annotation with Presence-Vector Classifiers
by Bertrand Le Saux and Giuseppe Amato
The interpretation of natural scenes, generally so obvious and effortless for humans, still remains a challenge in computer vision. Efficient image recognition software would lead to technological advances in many areas, from effective security to intelligent information retrieval. We propose a system that can classify new images on the basis of information learnt from a training set of previously annotated images.
An emerging research direction in the digital library field is the definition of services for discovering and manipulating knowledge. These include services to search for multimedia documents whose content is described by meta-data. The meta-data can be generated either manually (which is time-consuming but accurate) or automatically (with possibly lower costs but more questionable precision). Procedures for automatic meta-data generation can exploit techniques for image processing and visual feature extraction. Images could be automatically annotated through a visual-feature learning process. To do this, classifiers that can recognise semantic patterns associated with given categories of images are needed.
At ISTI-CNR, we have designed a system that can automatically label images by analysing their visual content. Principally, this involved the definition of an image representation appropriate for scene description and the design of a classification scheme to separate data in the image descriptor space.
Since the human description of visual content is often specific to an object or a given part of the image, image regions can be used to provide more semantic information than the usual global image features. We have developed an image description scheme that can identify which region types are present and which are not. First, the images from a training data-set are segmented into regions. These regions are then grouped into clusters according to their visual similarity, and the discrete range of possible region types that occur in the data-set is thus defined. For each image, a presence vector (indicating which region types are present) is built toprovide information on composition of the image. It is probable that a 'countryside' image would correspond to green foliage and dark ground regions.
The classifiers are built according to a two-step process. First, feature selection is used to estimate which region types are important to discriminate a given scene from others. This is done by mutual information maximisation. A simple implementation of a support-vector machine is then used to solve the margin-maximisation problem on the reduced presence vectors and consequently compute a decision rule to classify the images.
This approach has two advantages. First, it prevents over-fitting, by eliminating the less informative region types that can co-occur randomly with the concept being learnt and thus introduce noise into the classification. The second advantage is that the classifiers can learn the pattern of the scene composition more accurately when the useless image regions are not taken into account. This makes it possible to improve the performance of the predictor. The recognition rates reach more than 90% correctness, depending on the keyword being predicted.
We have tested the efficiency of our techniques on various image data-sets: generic ones for the recognition of natural scenes, and the databases of a press agency for topic-based labelling. In this second application, some news topics have been learnt and recognised, such as 'politics' or 'sport' (see Figure).
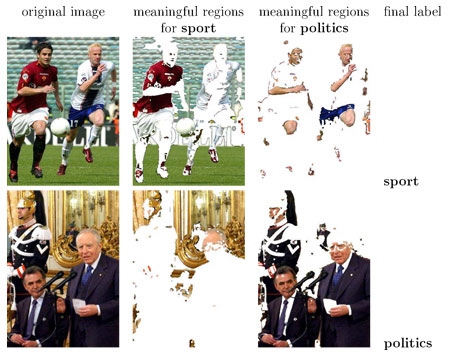 |
Meaningful regions used to recognize and discriminate between news topics. The original image is on the left, the following pictures show which image regions are considered as positive clues to classify the image according to the keywords 'sport' (the playground and the bright sport jerseys) and 'politics' (skin and dark-suit regions). |
The tools we have designed for automated image classification have been integrated into the MILOS multimedia content management system developed at ISTI-CNR, where they enhance its meta-data management and document retrieval capabilities. This research was supported by the Enhanced Content Delivery (ECD) project, funded by the Italian Government, and the Delos Network of Excellence, funded by the European Commission under the VI framework program. Future work will focus on improving the accuracy of the classifiers through a more precise description of the regions and the use of information about their spatial arrangement.
Please contact:
Bertrand Le Saux, ERCIM fellow at ISTI-CNR, Italy
Tel:+39 050 315 3139
E-mail: bertrand.lesaux isti.cnr.it
|